According to a report from Juniper Research, the global e-commerce fraud will reach $25 billion by 2024. With the increase in online banking, e-shopping and online insurance, fraud has become more common than ever. Fraud has become a major issue globally mainly for the e-commerce retailers.
To minimize the fraud machine learning plays a vital role. Machine learning is the science that is used for creating and applying algorithms that are capable of learning from the past using big data better and faster than humans ever will be able to. At the very beginning, machine learning collects data which is later used to analyze the data gathered extracting the required feature from it. Later, machine learning model receives training set which is used to predict probability of fraud. Finally, fraud detection model is created.
There are steps that are involved while working with the fraud detection algorithms. The first step is feeding data, where the data is fed into model, more the data better the model performs. The next process includes extracting features which is used to extract information associated. For payment transaction process it will check customer email address, mobile number and the PAN number if the customer applies for the loan. It will then check the location by tracking down IP address of customers to identify if there is any fraud. It then checks mode of payment—cards used for the transaction, name of cardholder and rate of fraud of bank account used.
Then we create fraud detection algorithm which is used for training the algorithm that is used to distinguish between ‘fraud’ and ‘genuine’ transactions. After training the algorithm we can create a model that is used for detecting ‘fraudulent’ and ‘non-fraudulent’ transactions in business.
Bhaktapur farmers start using harvesting machine
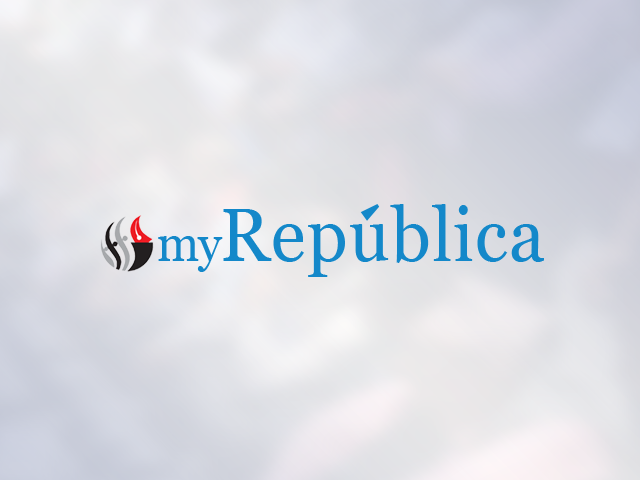
Machine learning algorithm types are used for fraud detection. They are supervised learning, unsupervised learning, semi-supervised learning, and reinforcement learning. On the supervised learning algorithm, we use labeled dataset for training model that is used to make prediction using data points with known outcomes. Supervised learning is divided into two parts a) regression where the output variable is continuous that includes algorithms like linear regression, dupport vector regression and poison regression and b) classification where the output variable is discrete. The popular classification algorithms are logistic regression, neural network, decision tree and naïve bayes classifier. The major drawback of the supervised model is that it’s not able to detect fraud that was not included on historical data. Unsupervised learning model is used to detect anomalous behavior. Here we don’t need to supervise the data as it discovers the useful information by use of principal component analysis and cluster analysis. This model continuously processes and analyzes new data and updates its models based on the findings. The unsupervised learning-based application utilizes clustering which is process of grouping data samples together into clusters.
Semi-supervised learning algorithm is a combination of supervised learning which uses labeled training data and unsupervised learning which uses unlabeled training data. It uses clustering process in-order to group distinct part and classification process in-order to identify data. Reinforced machine learning uses a technique called exploration/exploitation. In this process, action takes place, consequences are observed, and next action considers the result of the first action. It allows machine to automatically detect ideal behavior within a specified context. It is widely used in video games that provide flexibility to AI reactions for players through viable challenges. This learning technique is also used to amplify dialogue generation for chatbots and adjust natural language processing via relevant response according to user reaction. Most used reinforcement algorithms include Q-Learning, Monte-Carlo Tree Search and Asynchronous Actor-Critic Agents.
With the increase in fraud rate businesses all over the world have already started using data science. Machine learning is currently most used innovative tool to prevent fraudulent operations that lead to greater losses each year. Nepal has a lot to learn in this regard.